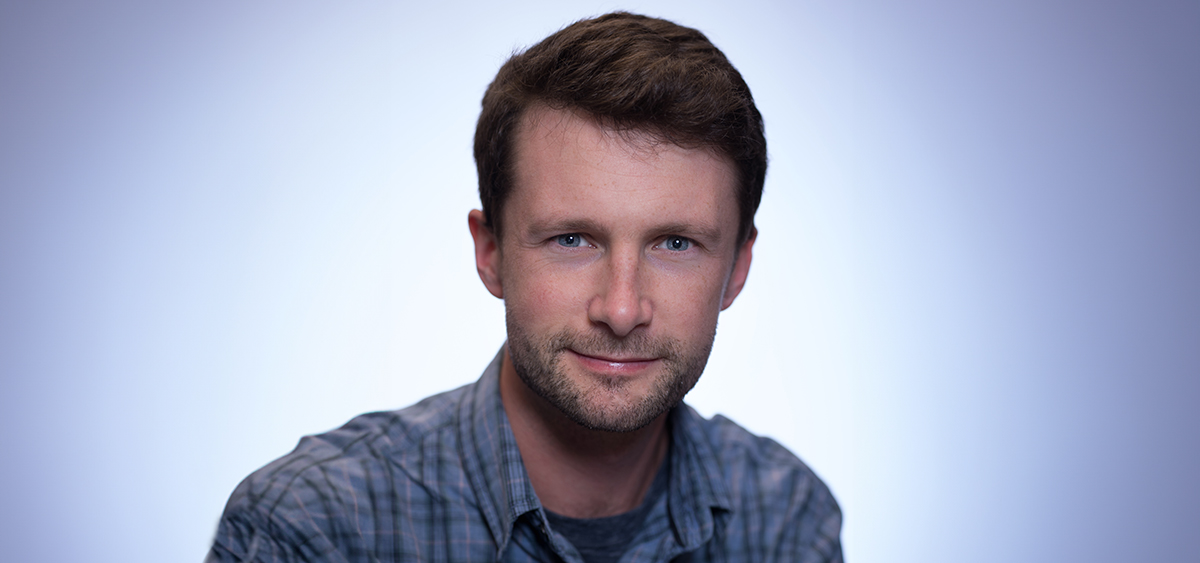
Sinai Health researchers have developed a novel computational workflow that promises to streamline the identification of cell types, potentially accelerating advances in personalized medicine and disease research.
In a study led by Dr. Kieran Campbell, Investigator at the Lunenfeld-Tanenbaum Research Institute, part of Sinai Health, the team applied machine learning, a type of artificial intelligence that recognizes patterns in vast amounts of data, to gene expression data obtained from individual cells.
“Our approach significantly accelerates the pace at which we can understand cellular diversity, which is critical for tackling diseases that are driven by rare but pivotal cell types,” said Dr. Campbell, who is also an Assistant Professor of Molecular Genetics and Statistical Sciences at the University of Toronto.
The journal Nature Communications published their findings.
Over the last few years, single-cell technologies have taken biomedical research by storm, enabling the measurement of gene expression in individual cells within a tissue. Since all cells in the body contain the same genes, these advances have enabled researchers to understand how cellular diversity is generated through changes in gene expression.
However, such high-dimensional data comes with challenges when it comes to data analysis. Researchers working with single-cell data often have to rely on manual annotation to be able to categorize cells into correct cell types.
To streamline analysis, in an effort led by PhD student Michael Geuenich, the team has evaluated a new method that combines two machine learning techniques, known as active learning and self-supervised learning. They found that this approach not only reduces the time and resources needed for cell type classification but also enhances the precision of these classifications, opening new doors for medical research and treatment strategies.
By making cell type annotation more efficient and accurate, the researchers aim to facilitate a deeper understanding of cellular behaviours and their roles in health and disease. For example, the method enables the discovery of rare cell populations, including those resistant to current therapies, potentially leading to the development of more effective treatments.
“This study represents a significant milestone in Sinai Health’s commitment to advancing machine learning and artificial intelligence research, and we’re excited about the impact it will have on biomedical research at large,” said Dr. Anne-Claude Gingras, Director of LTRI and Vice President of Research at Sinai Health.
This research was supported by funding from the Canadian Institutes of Health Research and the Natural Sciences and Engineering Research Council of Canada.