Meet Astir: The new AI-tool that can sort millions of cells in minutes
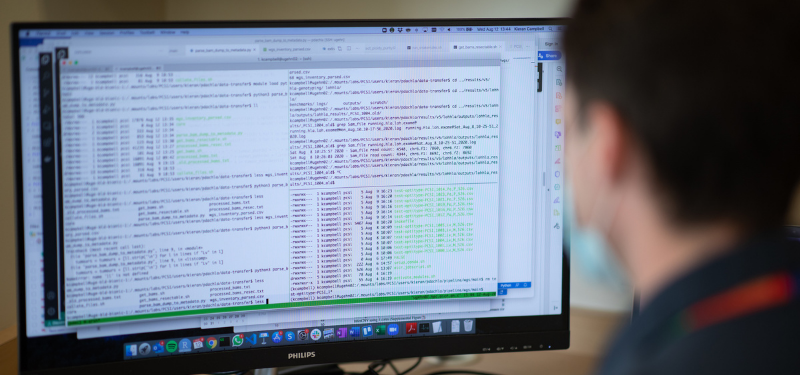
A new tool designed by Sinai Health researchers could one day help doctors make faster diagnoses and treatment decisions by using artificial intelligence to sort millions of cell types in the human body within minutes.
Scientists at the Lunenfeld-Tanenbaum Research Institute (LTRI) recently created Astir, a machine-learning method outlined in a new paper out today in the journal Cell Systems. Dr. Kieran Campbell, an investigator at the LTRI, said they used data from recently developed experimental methods to measure the protein content and location of a single cell in rapid time.
“One of the ways that Astir can be used is to perform automated quantification of cell types present in a patient’s tumour,” Campbell said. “Not only can it be used to form a diagnosis, but Astir could also help determine optimal treatment regimens for patients.”
Campbell said he hopes Astir will one day be used to assign cell types to patient samples in an automated fashion, ultimately allowing doctors to use the information as biomarkers to determine the optimal treatment for each patient in a truly personalized manner.
Astir is available publicly online, along with a tutorial with example data for researchers to familiarize themselves with the new tool.
The research was funded by grants from the Canadian Institutes of Health Research (CIHR), the Princess Margaret Cancer Foundation, NSERC, the Canada Research Chairs Program and the Canadian Foundation for Innovation.